Nvidia DGX Spark and DGX Station, redefining the Future of AI Workstations? Part 3/3
Making sense of Nvidia's latest Workstation announcements at GTC 2025 and exploring their impact on the workstation landscape.
Be sure you have checked:
Part 3: OEM Partnerships, Conclusions & Recommendations
The role of the OEM Partners: “Together we go far!”
It was interesting to see that the DGX Station (the golden tower) shown at the keynote was not present at the showcase. Wait, what? Yes — Nvidia chose to highlight the implementation of the DGX Station platform by the OEM partners, specifically Dell, HP & Asus. Showing partner designs “Time-To-Market” is an important sign of industry adoption and readiness.
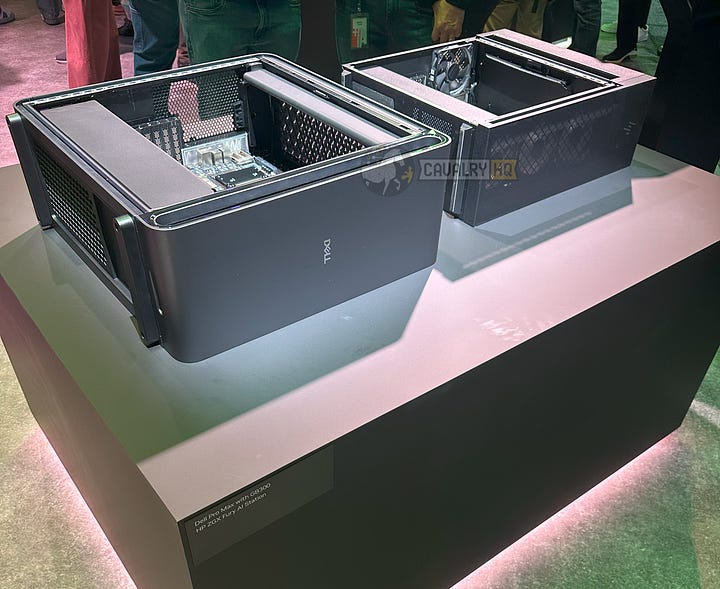
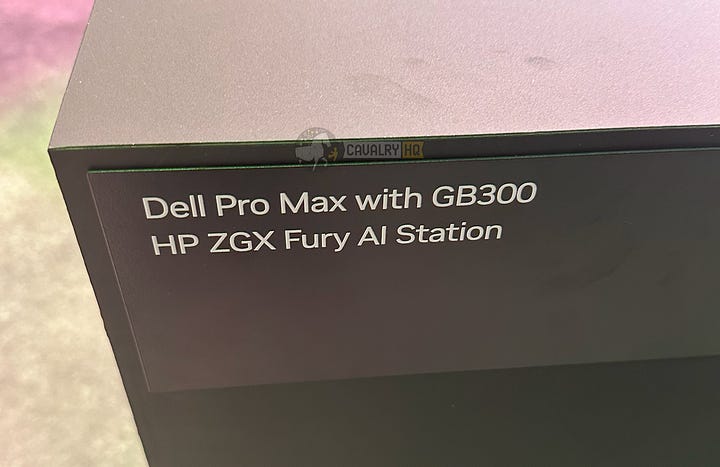
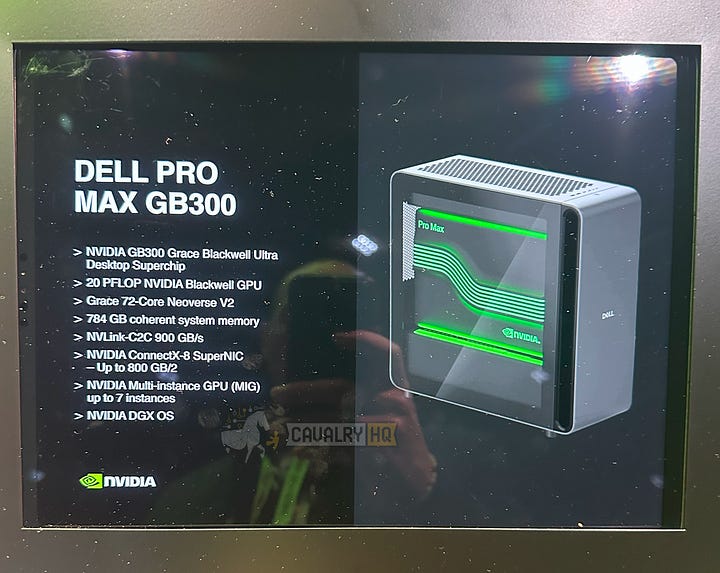
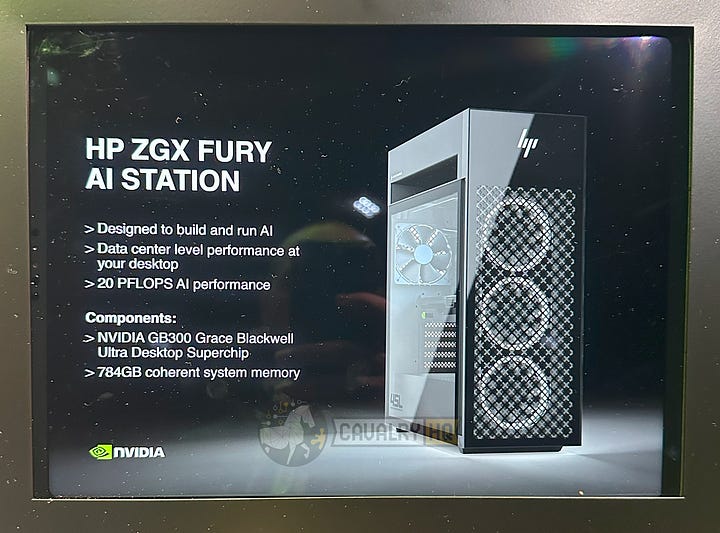
Nvidia also mentioned that other OEMs will follow, including BOXX, Lambda and Supermicro. The missing player: Lenovo. It will be interesting to see if they have a different plan or they will have a solution but are not ready to talk about it yet.
Just like there are DGX Station-based Workstations from partners, in their booths, there were DGX Spark-based Small-Form-Factor Workstations, again from Dell and HP. Great job by both companies being Time-To-Market! I expect that Lenovo will at least have a DGX Spark variant, the Lenovo ThinkStation P3 Ultra SFF is such a cool Intel+Nvidia machine, imagine it with an Nvidia GB10. Pure speculation at this point.
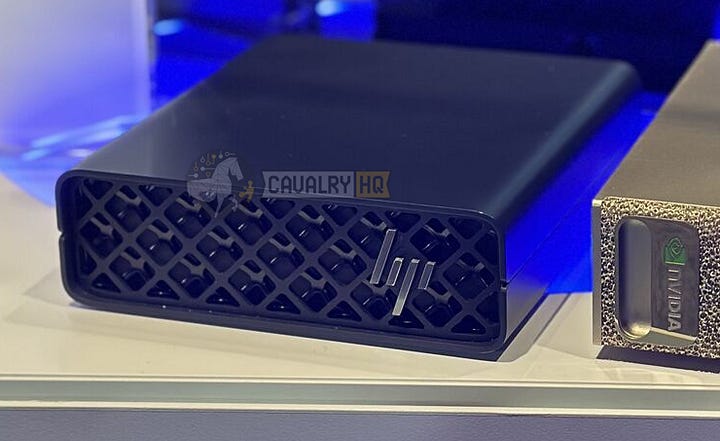
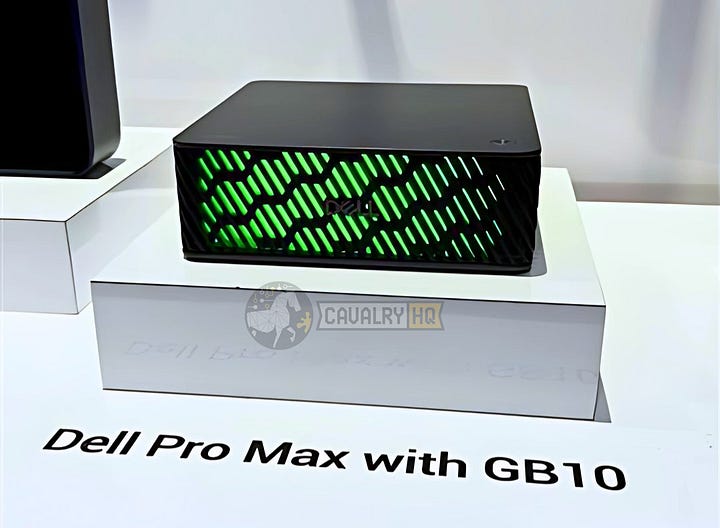
The Market Opportunity
So, with all this great technology, what do I think is the market opportunity here?
First, Nvidia has an opportunity with the Founder-Edition (please read: Nvidia-Branded systems). When a system is on a rack in the server room, maybe the looks don’t matter as much, but the DGX Spark and DGX Station are PC Clients, they will be on your desk and even if I’m not a fan of Gold-colored hardware, these systems are sure to call a lot of attention, be conversation starters and give developers bragging rights. Apple knows a lot about this.
Starting with the Mini PC (DGX Spark or OEM GB10 based), such prestige comes with a price premium of ~35% (extra ~US$1,000) versus what I expect from OEMs, based what I heard at the event and the Nvidia reservation website. The difference is actually smaller if you account for the BOM differences like storage, maybe other:
OEM implementations like the Dell Pro Max with GB10 (I think this is a placeholder name), and the HP ZGX Nano, will definitely be the volume runners, at least within the GB10-based options. It’s also great to see that Asus wants to keep growing in this space. Asus acquired the Intel NUC miniPC division, I’m sure such expertise has been very handy to bring the ASUS Ascent GX10 to market.
Are these systems here to compete with x86 SFF Workstations like the HP Z2 Mini or Dell Pro Max Micro? Based on current observations, that does not appear to be the case. This is a new category of SFF Workstation, it was positioned as a complement to your development laptop (or desktop). Can you use it on its own? yes, it has a display out, but I don’t think that’s the primary usage for it. Developers already have a development platform, typically a powerful laptop, there is less opportunity to try to replace such platform and instead try to “empower every developer” complementing whatever hardware platform they have.
There is more ROI for Nvidia to show the value of stacking multiple DGX Spark and I believe OEMs will be happier to sell multiple systems instead of cannibalizing one model with another.
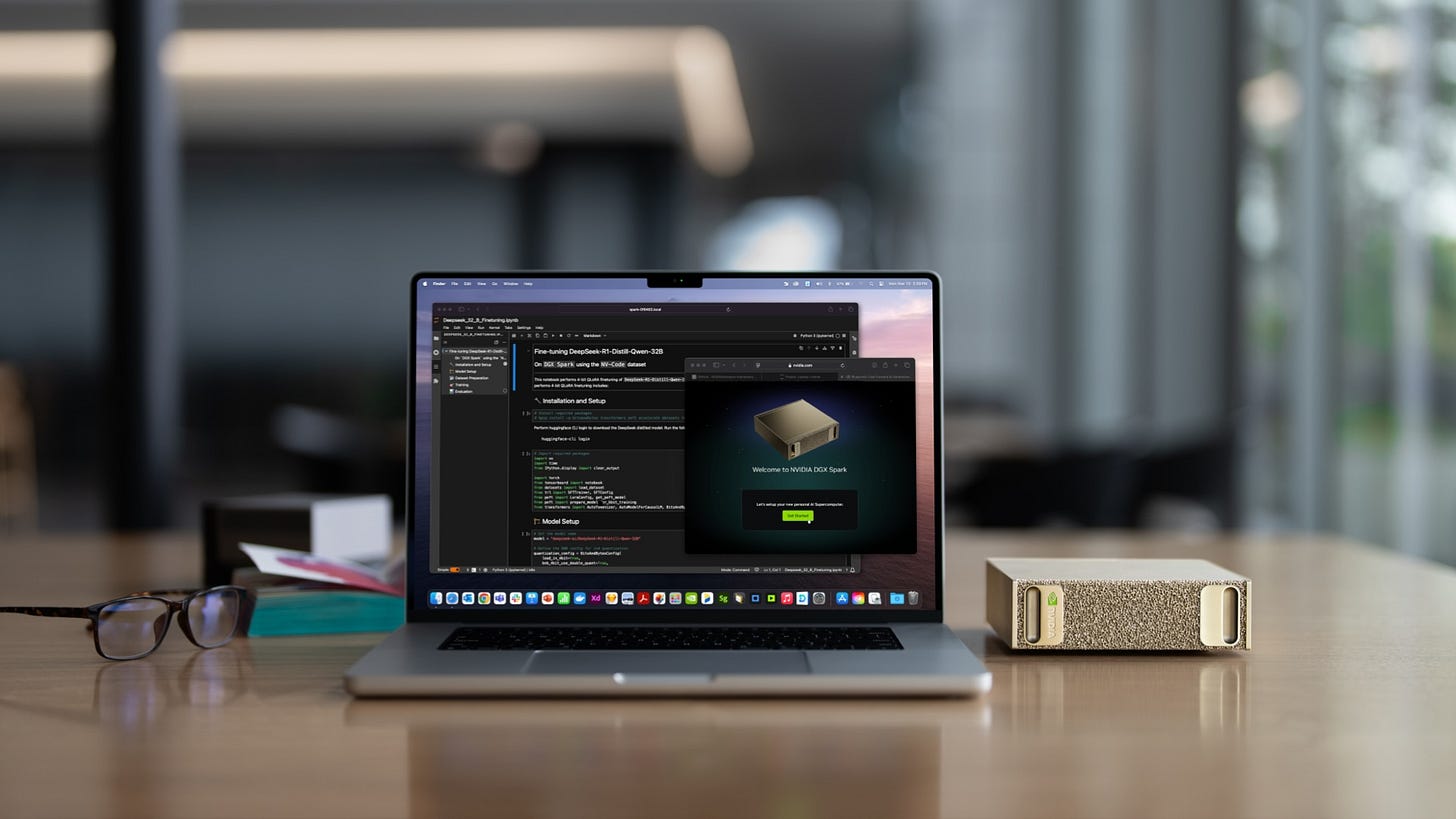
Talking about replacement, there is a potential change on the host machine, now with the separate accelerator. Until now, there have been a strong reason for the Data Scientist or A.I. developer to buy a very strong configuration with a very robust Nvidia GPU. Now with the DGX Spark, I think the user will be very happy to trade a less robust configuration (specially GPU-wise) for better battery life or form factor, since now you can rely on the Accelerated Compute that the DGX Spark offers, just need to remote in to harness such power. The most common development environment is browser based, according to JetBrain’s State of Developer Ecosystem Report 2024. This facilitates the adoption of the MiniPC as a compute accelerator. We will know more, when we know how easy it will be to setup your environment. I would recommend Nvidia to have not only very solid setup tools, but also good solutions, support and overall documentation. Easy setup is key to ramp this product; many developers will be sharing their experience as soon as they (we) get their (our) hands on one. It has to be robust on Day 0.
How much volume will Nvidia sell?
The volume of these new category of device is to be seen, there are opposite forces at play; on one side, these Small Form Factor (SFF) Workstations are not general-purpose machines, they are here for a reason: Data Science / A.I. Development. While the rest of x86-based Workstations are general purpose systems, for many usages and workflows, CAD being the #1 usage with >40% of volume last time I checked Jon Peddie Research’s Workstation Report (highly recommended). x86-based Workstations are also used in multiple industries, like Media & Entertainment, Finance, Energy, Healthcare and Life Sciences, etc. This suggests that the DGX Spark is here to serve a niche, but such niche is growing roots into all industries, so will its volume be limited?
On the other side, the optimistic side of me knows there is a massive number of developers that don’t code on a Workstation! Starting with but not limited to, developers that code on MacOS, but also developers with traditional commercial client notebooks or even gaming machines (don’t get me started). If only a tiny fraction of developers adds an accelerator like this to their environment, this can be a big win for Nvidia and OEM partners! Also, this is a great system for users starting to get deeper into A.I. It will be exciting to see this product line ramp up!
I know this will be controversial, but I think the closest thing to a competitor for the DGX Spark is the MacStudio. They are not the same thing; they don’t run the same tools and clearly the MacStudio doesn’t support all the Nvidia A.I. software ecosystem. Having said that, both are small form factor desktops with Unified Memory space and powerful GPUs to actually do something with the data. Purists will rightfully call me crazy, but I have seen creators like Alex Ziskind running LLMs on a Mac Studio, quite successfully.
Enough talk about knives, let’s talk about Samurai/katana level swords! The DGX Station will easily cost 5-digits, I think if you even try to buy the 496GB of RAM memory for the CPU it would cost you a lot, let’s not even talk about the 288 GB HBM3e for the GPU.
Now, I’m not saying that paying a lot for a DGX Station is a bad deal, quite the opposite. Remember those “A.I. Workstations” that the OEMs have been selling since 2020? I think that is where there is potential for cannibalization of the market. In order to get 288GB of Memory for those advanced LLMs, you would need, oh wait, you can’t get that much with RTX Ada Generation, you are limited to 4x GPUs on a Workstation like the Dell Precision 7960, an HP Z8 Fury or a Lenovo PX, so are capped at 192GB of GPU GDDR7 memory after paying ~$25,000 in GPUs. The New Nvidia Blackwell-based RTX Pro 6000 have a very desirable 96GB DDR7 per card, so 3x GPUs would do the trick. Having said that, the HBM3e memory on DGX Station should be more expensive, yet higher bandwidth, more power efficient and lower latency vs GDDR7, so for A.I. Development, the DGX Station has a lot of advantages. And that is just the memory part of the equation, when it comes to compute, each RTX Pro has 4,000 (3x = 12,000) TeraFLOPs vs the 20,000 TeraFLOPs of the DGX Station.
I have seen vendors claiming that because their iGPU can address a lot of memory, they are good for A.I. but there is a balance between compute and memory, just because you can put a lot of food in your mouth, doesn’t mean that you can chew, swallow and digest it all. Think of it like a production line, but that is a topic for another day.
Conclusions + Recommendations to AMD, Intel & OEMs
First of all, THANK YOU for making it all the way here and walking with me until the end. Being my first deep down on CavalryHQ, I didn’t put this report behind a paywall, I kindly ask you to subscribe by entering your email below and supporting this effort in any way you can; if you can’t become a paid subscriber, becoming a free one or sharing with your stakeholders are greatly appreciated.
To show you my appreciation, here you have a bowl 🥣 of M&Ms (🔴🟢🟡🔵🟠) with the brown ones removed (🟤🟤) :-D (if you didn’t get it, check the last Reference below) Thanks for being here!
The Winners 🏆🥇🥈🥉
Of course, Nvidia, empowering developers with the most powerful GPU or multiple GPUs on the Intel-based Workstations, AMD-based Workstations and now giving Mac users a great way to add 100% Nvidia (CPU+GPU) to their setup!
OEMs also win, especially Dell, HP & Asus, if they keep working close to Nvidia, they can be very successful if this product line ramps up. Also, OEMs can now sell systems to Mac users, when was the last time you saw that? that is the textbook definition of TAM Expansion!
Most importantly, I think the Users are the biggest winners, these new systems will unleash their productivity with some upfront cost, but potentially lower TCO. Save in Cloud Credit’s cost early on, when exploring, experimenting and designing. Then, when ready, your code will be ready to deploy on an Nvidia-based cloud instance.
The Challenge: Differentiation
OEMs need to find ways to differentiate. When you have a platform with an Nvidia CPU, GPU, that also has soldered memory, I started to wonder, how will they differentiate? I assume everyone had exactly the same motherboard at GTC because of how new the platform is, I hope each OEM can have their own design, add more m.2 slots, PCIe ports, different thermal solutions, better software tools. Having said that, will there be enough volume to justify multiple designs? I’ll keep an eye on this as the launches and availability happen later this year. There are other non-technical ways to differentiate via bundling with other products, or technical ones like software tools to assure an easy and graceful transition when the accelerator (i.e. miniPC) is Online/Offline. I have a handful of other ideas that I’ll share with the OEMs when I have a chance to meet with them.
I challenge the OEM teams to use these systems internally as much as possible, this is a new way to work, and bridges will have to be built to assure smooth workflows and happy users. First one to figure this out will be rewarded in the market.
At risk
The x86 CPU vendors: Intel and AMD. Seeing Workstations with Nvidia GPUs is the norm, and GPUs do take a lot of the Share-of-wallet, but with Nvidia CPU and GPU should be a red flag to anyone paying attention inside these companies. If this product line ramps well, it will take time for the finance departments at Intel and AMD to notice that something is going on; initially it will feel like there is market softness, when in reality it is the effect of market cannibalization; a couple of years later, some market decline, by the time they realize what is really happening, it will be too late. Their time to act is now.
Recommended Action #1, call IDC and ensure they have a way to track this line up on the quarterly IDC Workstation Report. Same goes for Jon Peddie Research. The Workstation Market has very few consumption reports and, if this line up is not properly tracked, Intel and AMD will fight with a stealth competitor, that is already ahead in Share-of-Voice and Share-of-Compute. Only Jean-Claude Van Damme can win a fight while blind1 ;-)
Recommended Action #2, start measuring Share-of-Compute on key workflows in the biggest industries: Engineering (Product Design and Manufacturing and Architecture, Engineering and Construction) and Media & Entertainment (3D, VFX and Video/Audio Production). Only focusing on the workloads that run exclusively on CPU will blindside you on how relevant each of your products are to the power users in such industries. This relevance information is nice-to-have for the OEMs, but to Intel and AMD is way more critical to understand. How many workloads look like the SideFX Houdini example I shared on Part 1? What are other examples of the opposite balance where CPU is key? analyzing this is part of the CavalryHQ’s mission, reach out if you want to collaborate with me to understand this.
Let’s continue the conversation
Can I help you bring these products to market? Would you like an in-depth review of your latest Workstations?
Do you want an independent voice on your next salesforce training or webinar?
Do you need help selecting the best Workstation solutions for your company?
I’m one click away…
References:
Nvidia DGX Station (2018): White Paper: https://images.nvidia.com/content/newsletters/email/pdf/DGX-Station-WP.pdf
Nvidia DGX Station (2018): Data Sheet: https://www.nvidia.com/content/dam/en-zz/Solutions/Data-Center/dgx-station/dgx-station-print-explorer-datasheet-letter-final-web.pdf
Nvidia DGX Station A100 (2020): Data Sheet: https://www.pny.com/en-eu/File%20Library/Professional/DATASHEET/DGX/DGX_Station_A100_Datasheet_PNY-WEB.pdf
Nvidia DGX Spark: https://www.nvidia.com/en-us/products/workstations/dgx-spark/
Dell Blog - Nvidia GTC coverage: https://www.dell.com/en-us/blog/pushing-boundaries-driving-ai-innovation-at-every-scale-with-dell-pro-max/
No Brown M&Ms reference: The Truth About Van Halen And Those Brown M&Ms : The Record : NPR
Bloodsport: https://www.imdb.com/title/tt0092675/?ref_=nv_sr_srsg_0_tt_7_nm_1_in_0_q_bloodsport